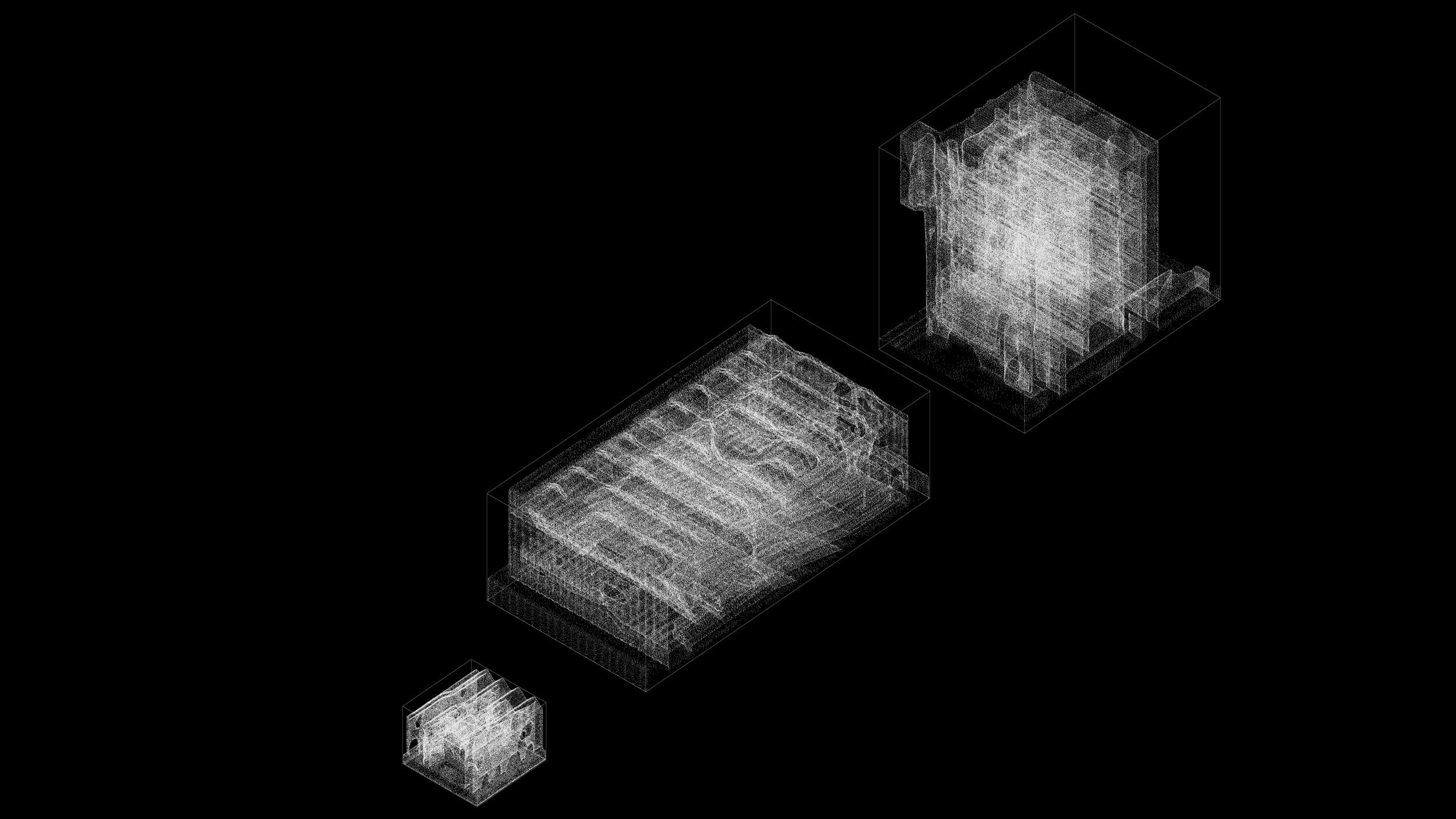
SECTIONAL SAMPLING
Controlled Generation of High-Resolution Architecture Design
This paper addresses the challenge of algorithmically generating 3D architectural designs. Conventional rule-based methods and GAN-based 3D generative models offer limited extrapolation and control and are limited to generating low-resolution results. Our method utilizes fine-tuned latent diffusion models, specifically Stable Diffusion, applied to an architectural dataset, to produce detailed architectural sections. These are then synthesized into 3D models using latent interpolation.
The pipeline starts with creating sectional poché datasets for various building typologies—houses, museums, and offices. The fine-tuned Stable Diffusion models, enhanced through LoRA, generate new sections, conditioned on section profiles via ControlNet. This ensures fluid transitions between sections. The generated sections are reconstructed into 3D models using the Marching Cubes algorithm within the Grasshopper. Our research contributes significantly to AI-generated architectural content, providing designers with advanced tools for creating high-quality, controlled models during the initial stages of a project.
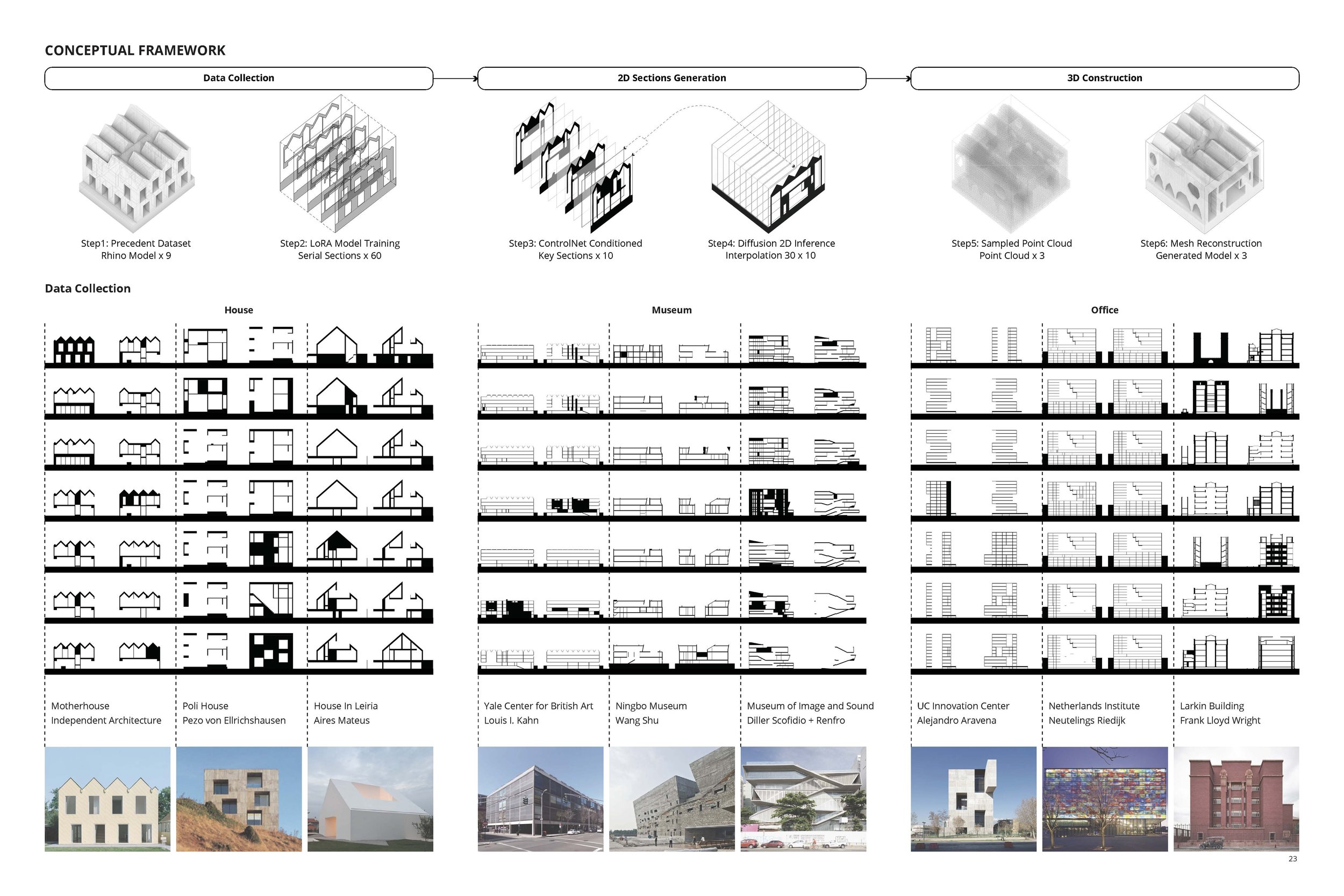
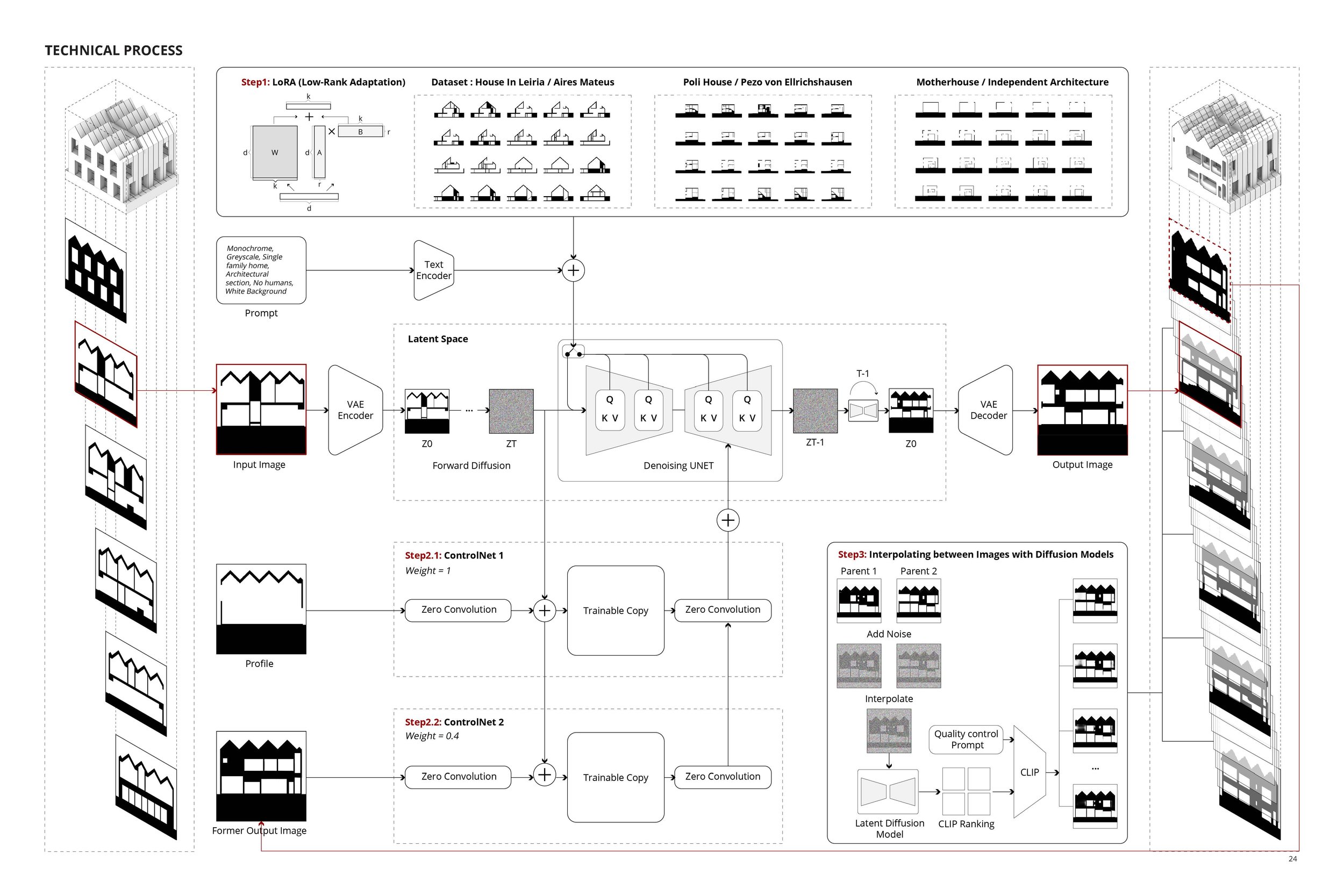
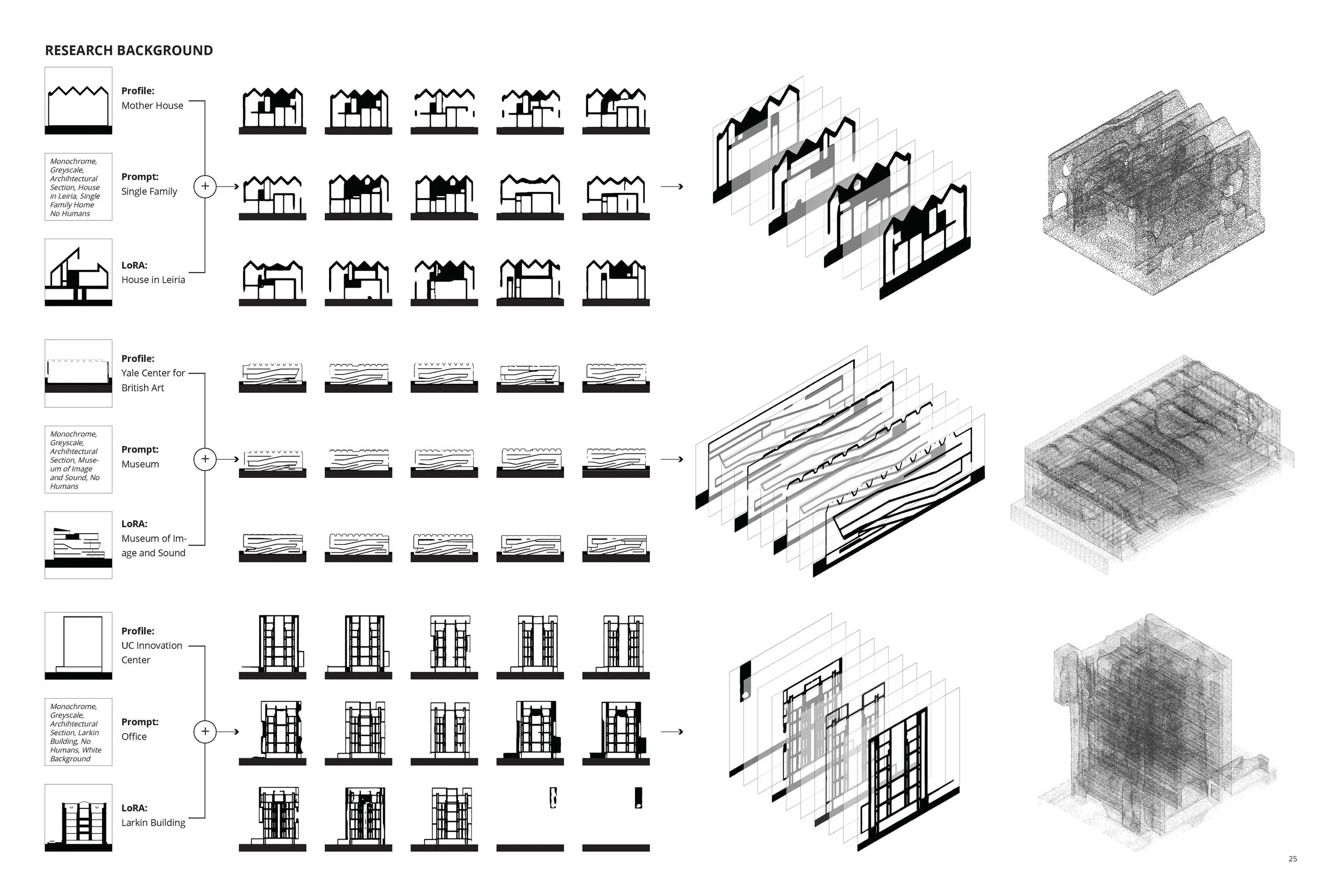
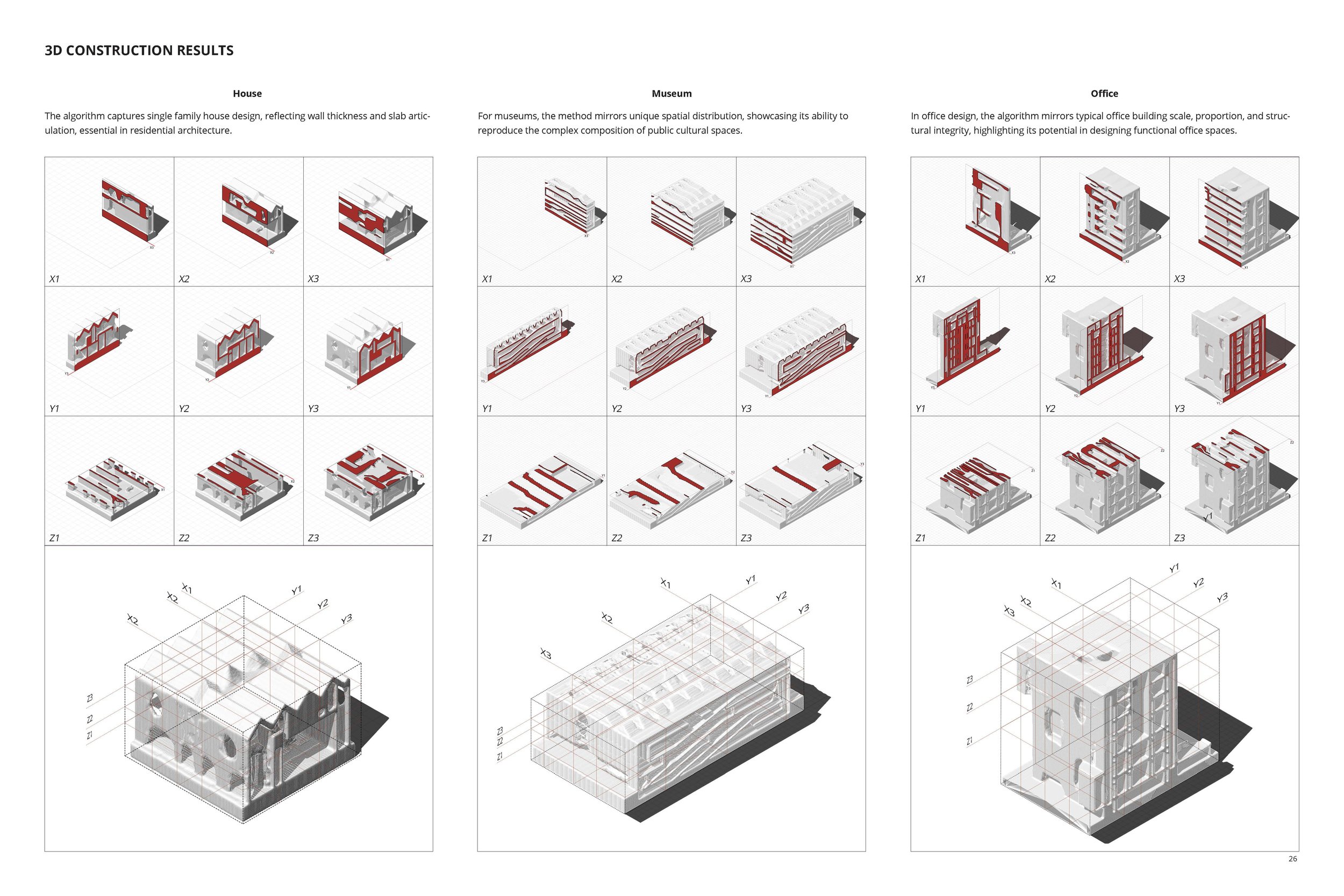